Stable Diffusion
Stable Diffusion is a latent text-to-image diffusion model capable of generating photo-realistic images given any text input. It's created by researchers and engineers from CompVis, Laion, and Stability AI.
Stable Diffusion Version v1.x
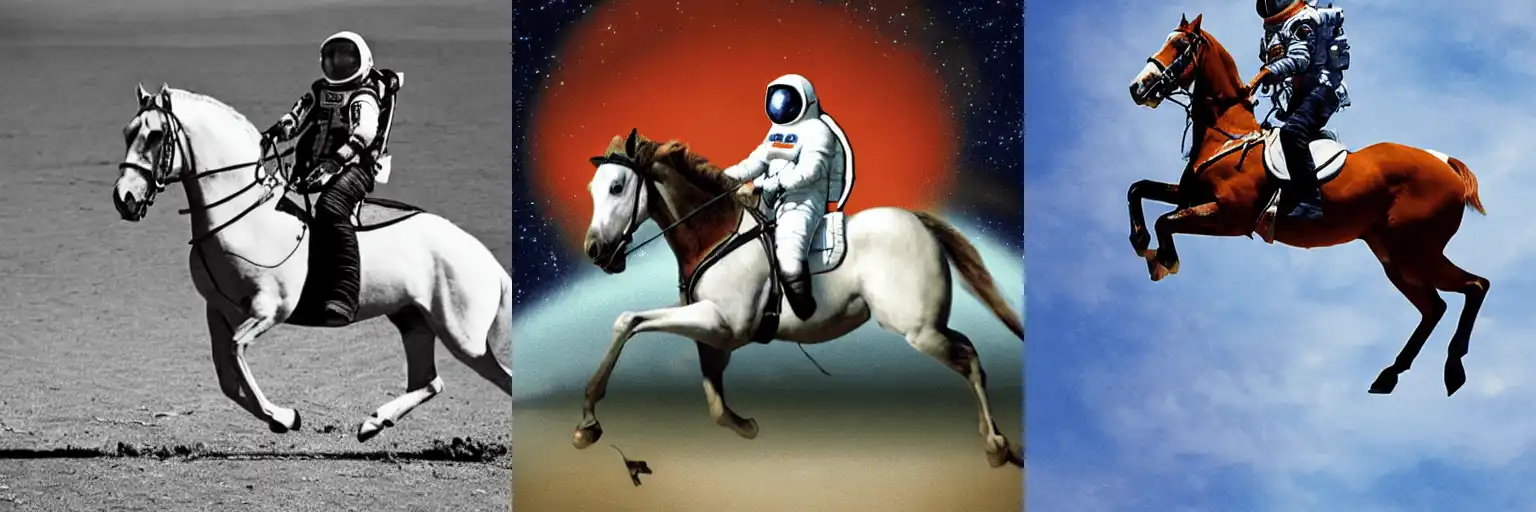
For the first version 5 model checkpoints are released. Higher versions have been trained for longer and are thus usually better in terms of image generation quality then lower versions. More specifically:
- stable-diffusion-v1-1: The checkpoint is randomly initialized and has been trained on 237,000 steps at resolution
256x256
on laion2B-en. 194,000 steps at resolution512x512
on laion-high-resolution (170M examples from LAION-5B with resolution>= 1024x1024
). - stable-diffusion-v1-2: The checkpoint resumed training from
stable-diffusion-v1-1
. 515,000 steps at resolution512x512
on "laion-improved-aesthetics" (a subset of laion2B-en, filtered to images with an original size>= 512x512
, estimated aesthetics score> 5.0
, and an estimated watermark probability< 0.5
. The watermark estimate is from the LAION-5B metadata, the aesthetics score is estimated using an improved aesthetics estimator). - stable-diffusion-v1-3: The checkpoint resumed training from
stable-diffusion-v1-2
. 195,000 steps at resolution512x512
on "laion-improved-aesthetics" and 10 % dropping of the text-conditioning to improve classifier-free guidance sampling. - stable-diffusion-v1-4: The checkpoint resumed training from
stable-diffusion-v1-2
. 195,000 steps at resolution512x512
on "laion-improved-aesthetics" and 10 % dropping of the text-conditioning to improve classifier-free guidance sampling. - stable-diffusion-v1-5 checkpoint was initialized with the weights of the Stable-Diffusion-v1-2 checkpoint and subsequently fine-tuned on 595k steps at resolution 512x512 on "laion-aesthetics v2 5+" and 10% dropping of the text-conditioning to improve classifier-free guidance sampling.
- stable-diffusion-v2 model is resumed from stable-diffusion-2-base (
512-base-ema.ckpt
) and trained for 150k steps using a v-objective on the same dataset. Resumed for another 140k steps on768x768
images.
More details about stable diffusion v1-1 - v1-5
Stable Diffusion Version v2.x
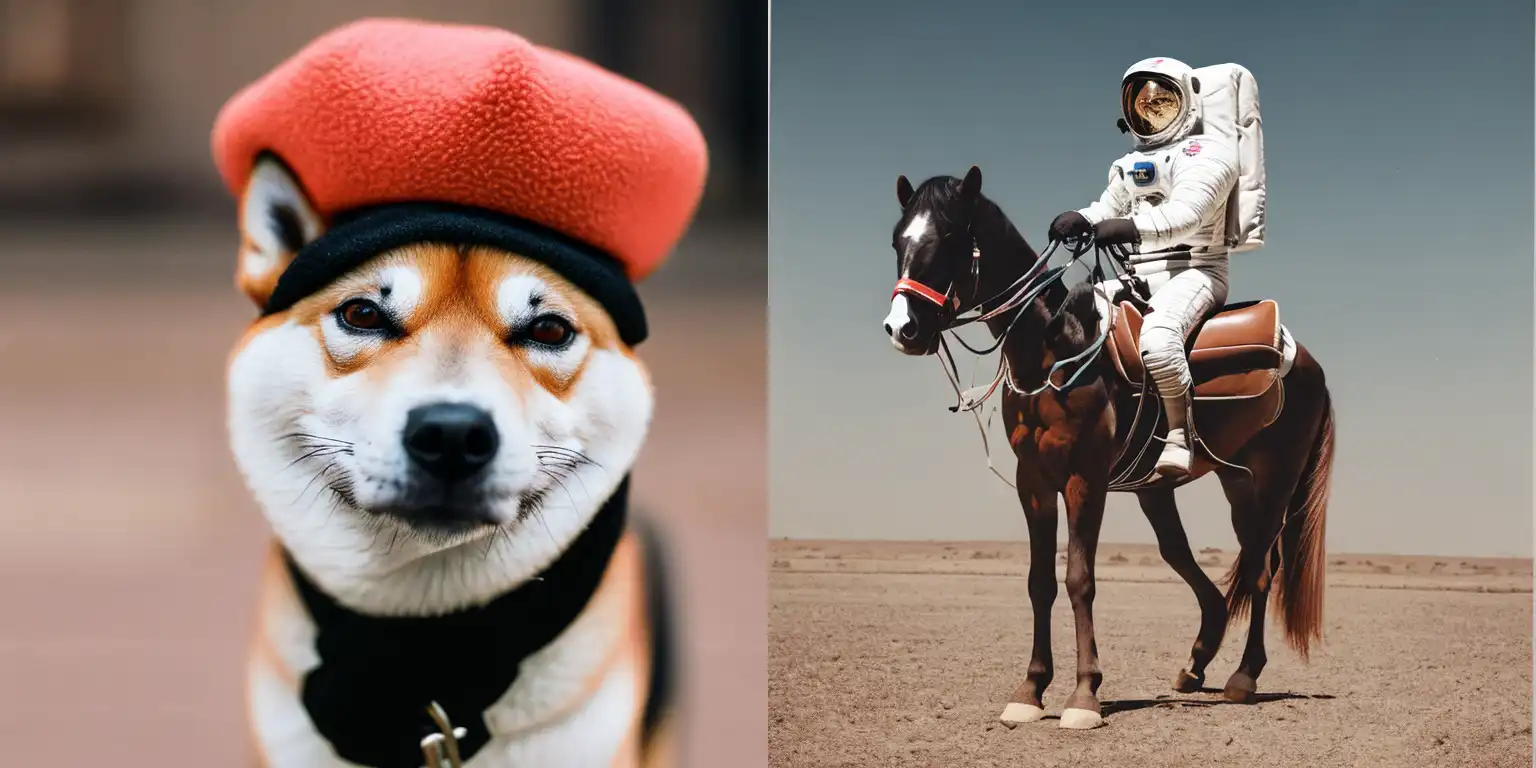
- stable-diffusion-v2 model is resumed from stable-diffusion-2-base (
512-base-ema.ckpt
) and trained for 150k steps using a v-objective on the same dataset. Resumed for another 140k steps on768x768
images. - stable-diffusion-2-1 model is fine-tuned from stable-diffusion-2 (
768-v-ema.ckpt
) with an additional 55k steps on the same dataset (withpunsafe=0.1
), and then fine-tuned for another 155k extra steps withpunsafe=0.98
.
More details about stable diffusion v2 - v2-1
Stable Diffusion XL
Stable Diffusion XL (SDXL) was proposed in SDXL: Improving Latent Diffusion Models for High-Resolution Image Synthesis.
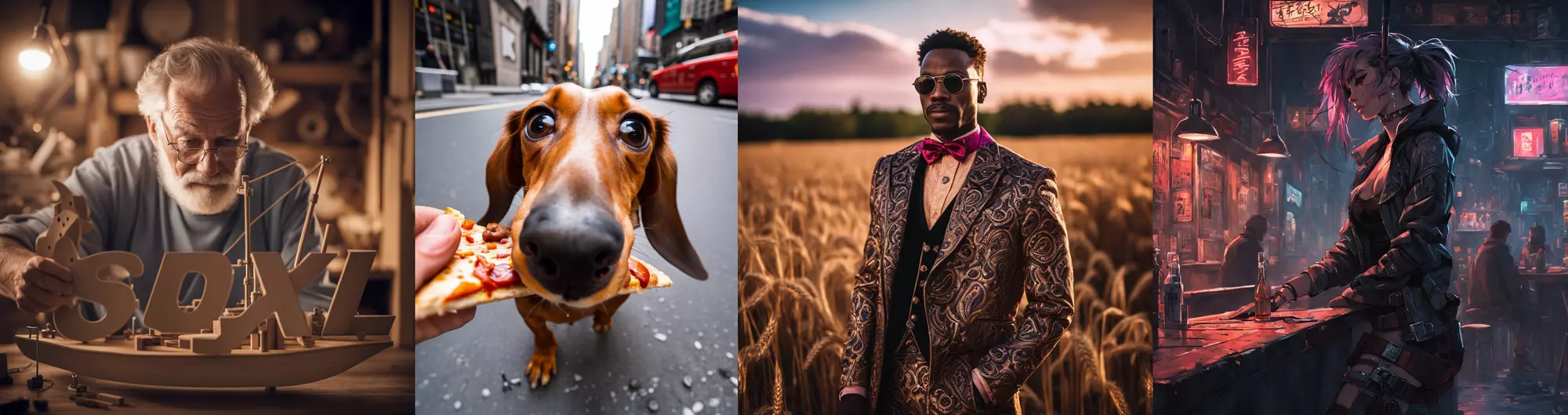
Compared to previous versions of Stable Diffusion, SDXL has made improvements in 3 areas.
Improvements:
- Multiple conditioning schemes: Different ways to manipulate the model's output based on text prompts.
- Training on diverse aspect ratios: Enables generating images in various shapes and sizes.
- Refinement model: Enhances the visual quality of generated images after initial creation.
Results:
- Drastically improved performance: Compared to previous Stable Diffusion models.
- Competitive with existing state-of-the-art: Produces results comparable to top image generation models.
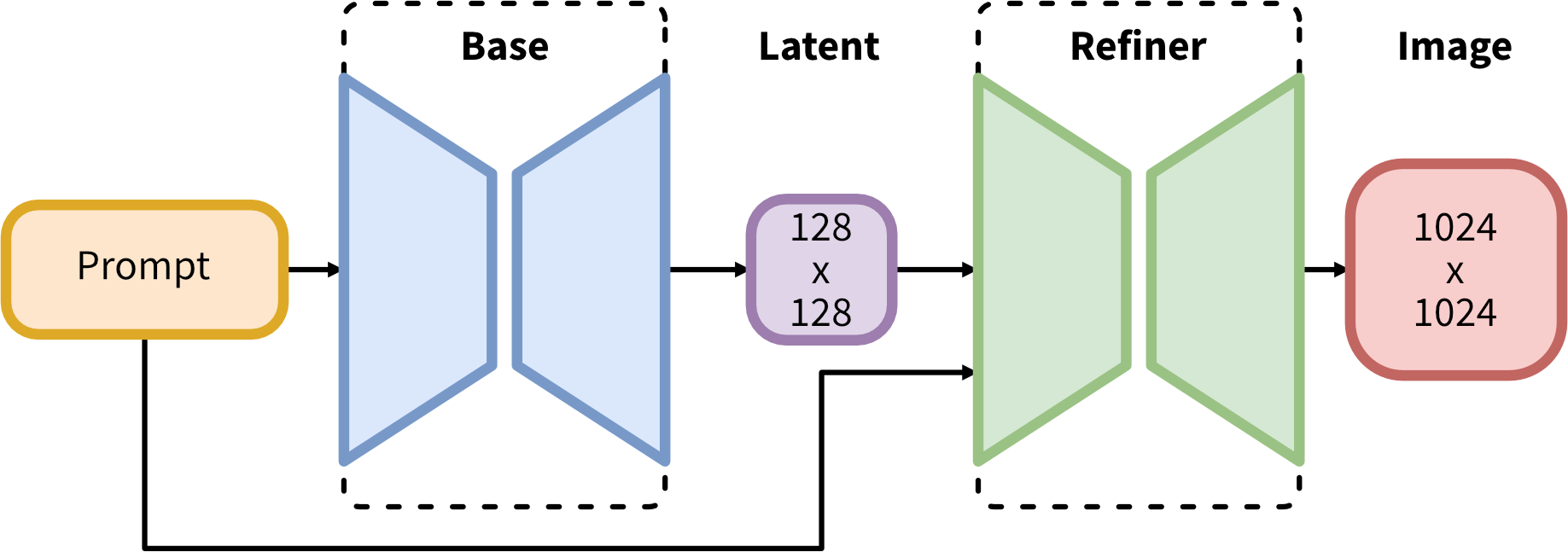
More details about stable diffusion XL
Which one should I use
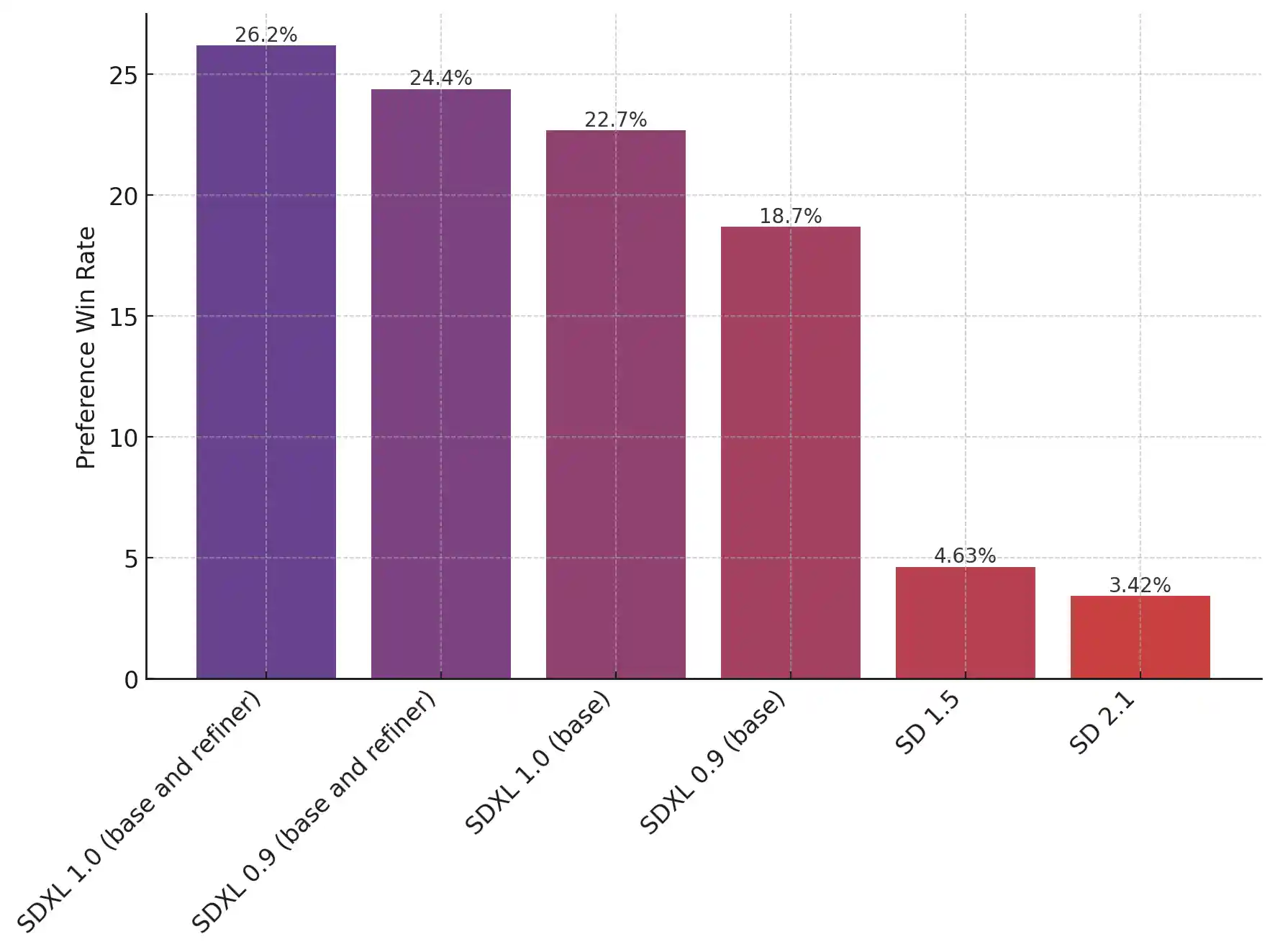
The chart shows that users strongly favor SDXL over its ancestors, especially when enhanced with the refinement technique. While the basic SDXL outshines older models, it's the refined version that truly steals the show.